Crystallography, cryo-EM and AlphaFold: a perfect trio sheds light on key human antibacterial proteins
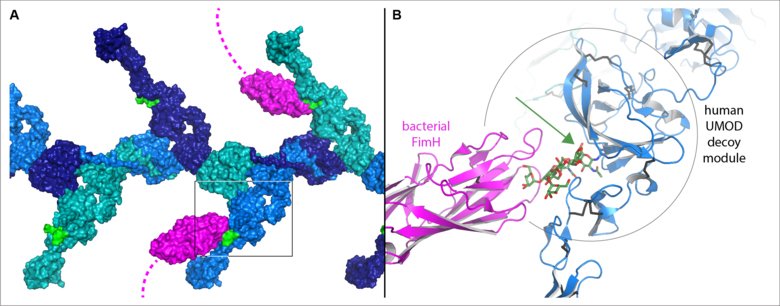
A team led by Karolinska Institutet has combined Artificial Intelligence (AI) with structural biology to gain insights into two similar proteins that prevent bacterial infection in the urinary tract and the gastrointestinal system. Their results are published in "Nature Structural & Molecular Biology".
"These findings could in principle be explored to develop a decoy alternative to conventional antibiotic treatments. And our structural work explains many human mutations associated with kidney diseases, as well as provides information on a protein region whose interaction with filtered light chains is implicated in cast nephropathy”, says Professor Luca Jovine at the Department of Biosciences and Nutrition and corresponding author of the paper.
Uromodulin (UMOD) is a protein whose filaments play an important role in preventing urinary tract infection, the most common form of non-epidemic bacterial infection that affects about 150 million people per year. It carries a special sugar chain that is not modified like others and remains of high-mannose type. This acts as a decoy by mimicking high-mannose sugar chains on the surface of the urinary tract, to which bacteria would normally attach using a sugar-binding protein called FimH at the tip of their hair-like appendages. Instead, the bacteria bind to the sugar chain of UMOD and are eventually removed through the urine. During recent years, evidence has shown that glycoprotein 2 (GP2), a UMOD-like molecule produced in the pancreas and the intestine, plays a similar role in counteracting bacterial infections in the gastrointestinal system.
“We wanted to determine which sugar chain of GP2 corresponded to the high-mannose chain of UMOD and understand how the proteins manage to keep these sugars ‘special’ so that they act as a bait for bacteria” explains Luca Jovine.
AI positioning itself as a tool in structural biology
In collaboration with Daniele de Sanctis at ESRF synchrotron in Grenoble and Marta Carroni at SciLifeLab in Stockholm, the team managed to collect X-ray crystallographic and cryo-electron microscopy (EM) data on GP2 and UMOD, respectively. However, different technical issues complicated its interpretation. It was at this point that the scientists began a collaboration with DeepMind and the team behind AlphaFold - a machine learning program that attracted significant attention from the structural biology community based on its performance in the 14th Critical Assessment of Structural Prediction competition. It turned out that, although AlphaFold had never “seen” UMOD or GP2 before, its predictions were extremely good. This allowed the team, which also included Bin Wu at Nanyang Technological University in Singapore and Nao Yamakawa at Lille University, to both make sense of the GP2 X-ray information and fit low resolution cryo-EM maps of UMOD.
The work revealed that the functionally crucial decoy module of UMOD and GP2 consists of two parts separated by a crevice. Although the sugar chains that bind bacteria are attached to different regions of the GP2 and UMOD sequence, the 3D structures show that, in both cases, their base is positioned within the crevice. This explains how these specific sugar chains are shielded from modification and can thus presented as hooks for fishing pathogenic bacteria (Figure 1).