Artificial intelligence takes structural biology to the next level
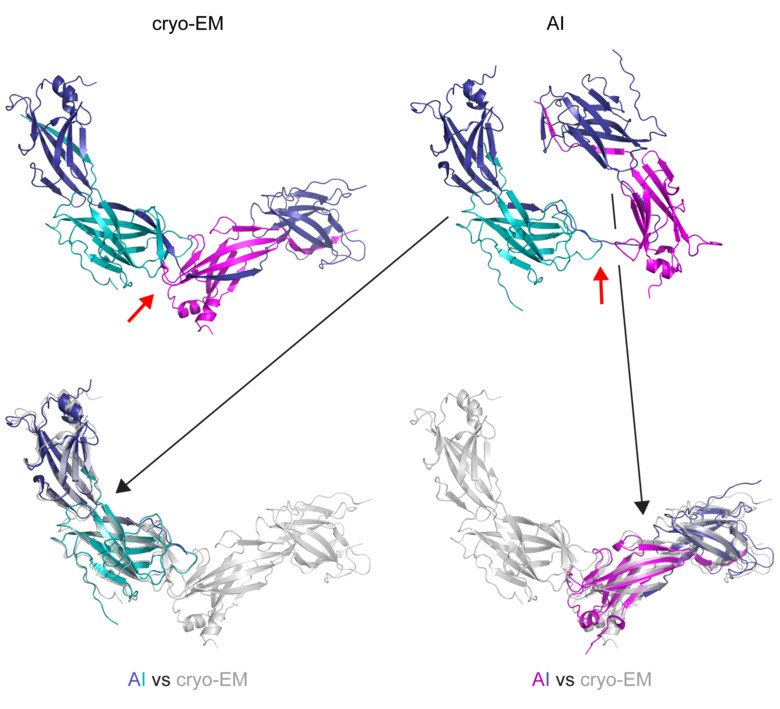
A scientist at Karolinska Institutet reports that machine learning can be used to gain insights into molecular events that change the shape of proteins after they are made, regulating their ability to interact with each other. This suggests that artificial intelligence (AI) may allow us, in the future, to accurately simulate highly complex biological scenarios in silico as well as leverage this information for therapeutic intervention.
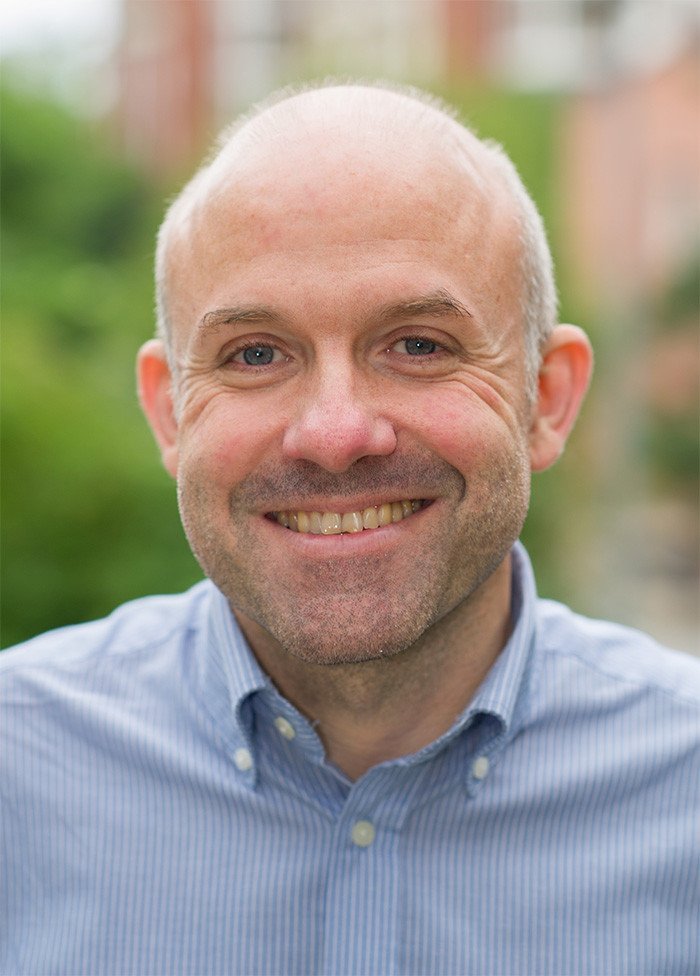
Detailed knowledge of the three-dimensional (3D) structure of proteins is essential to understand their function, assess the impact of human pathogenic mutations and help the rational design of new drugs. Recently, a machine learning program, AlphaFold2, was shown to be able to predict this 3D information from protein sequence alone with near-experimental accuracy. Now, professor Luca Jovine, at the Department of Biosciences and Nutrition, Karolinska Institutet, reports that AlphaFold2 can also be applied to the study of molecular events that modify the shape of proteins after they are synthesized, regulating their interaction with other molecules.
“This work is important because it brings support to the idea that the computational approaches underlying AlphaFold2 and related programs, such as RoseTTAFold, have potentialities that extend well beyond the high-accuracy prediction of individual protein structures”, says Jovine.
AI as an approach in structural biology
Determination of the 3D structure of proteins has traditionally been a long, difficult and expensive process that depended on experimental techniques such as X-ray crystallography, nuclear magnetic resonance (NMR) and, more recently, cryo-electron microscopy (EM). Against this background, the development of machine learning tools such as AlphaFold2 has been immediately recognised as a momentous advance that is bound to revolutionise structural biology. However, the application of AI to more complex structural problems, such as for example understanding how proteins interact with each other to form complexes, remains largely unexplored.
In the study, the first of this kind to be published from Sweden, Jovine shows that AlphaFold2 can reproduce a significant part of the complex molecular re-arrangements that take place when uromodulin, a protein that protects our body from urinary tract infections, assembles into filaments after being cut by another molecule. Considering that AlphaFold2 had no knowledge of the uromodulin filament structure, this surprising result suggests that the same basic approach could be generally applicable to many other biomedically important molecular systems, whose function is similarly regulated after protein synthesis.
Using machine learning to study protein-protein interactions: From the uromodulin polymer to egg zona pellucida filaments.
Jovine L
Mol Reprod Dev, 2021