Prostate cancer management: towards personalization with AI-assisted pathology and improved active surveillance
Prostate cancer is a major global health concern and is the most common cancer-related cause of death in Sweden. Prostate cancer screening using PSA reduces prostate cancer mortality but also leads to significant overdiagnosis and overtreatment of low-risk cancers. The pathological evaluation of prostate biopsies determines the therapeutic course of treatment for prostate cancer patients.
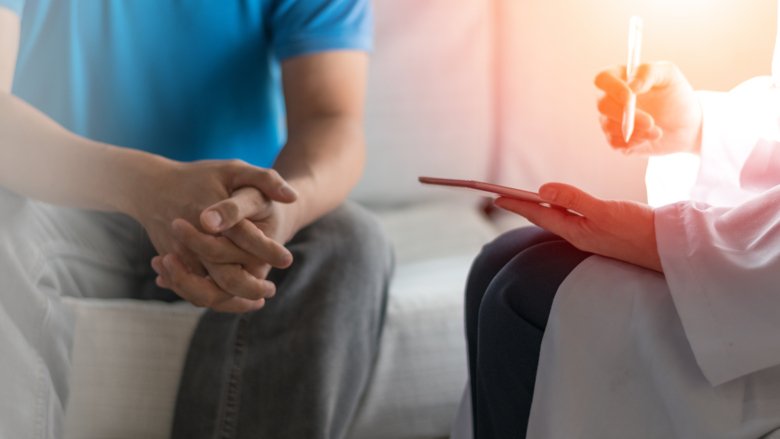
However, current clinical practice faces challenges such as a high level of inter-observer variability among pathologists and a global shortage of uro-pathologists. Therefore, improved risk stratification and effective active surveillance are crucial to balancing the benefits of screening with the risk of overdiagnosis and overtreatment.
In his thesis, Henrik Olsson at the Department of Medical Epidemiology and Biostatistics, focused on improving active surveillance management and developing prediction models for prostate cancer diagnostics.
What are the most important results in your thesis?
Active surveillance aims to reduce overtreatment and potential treatment-related side effects and is increasingly utilized as a safe alternative to curative treatment for low-risk prostate cancer. A drawback with current active surveillance is the large number of prostate biopsies the men undergo, which leads to reduced adherence to follow-up biopsies and increased risk of infections and other complications. In my first study, we found that most men that enrolled in active surveillance remained on surveillance, but they did not receive a follow-up in line with the guidelines when it came to repeat biopsies and PSA tests. We conducted the STHLM3-AS trial to assess a less invasive way to detect clinically significant prostate cancer in active surveillance including the so-called Stockholm3 prostate cancer test and MRI-targeted biopsies. In conclusion, the results from our study suggest that combining systematic and MRI-targeted biopsies in active surveillance increase sensitivity to detect prostate cancer upgrading in these men. The inclusion of risk prediction models in active surveillance may decrease the requirement for MRI use in patients with low-risk prostate cancer.
We have developed artificial intelligence (AI) for prostate cancer diagnostics. The results show that computational pathology can assist pathologists in prostate cancer diagnostics with high accuracy, which has the potential to reduce inter-observer variability and provide high-accuracy diagnostics in parts of the world where there is a shortage of prostate pathology specialists. However, more work is needed before we have mature AI systems implemented clinically. In particular, the generalizability of AI systems is unsolved. The appearance of digitized biopsies varies greatly in terms of different tissue preparation techniques and digital scanners used in different clinics. We therefore also developed conformal prediction to estimate the uncertainty of the predictions by the AI model. The conformal predictor was able to detect unreliable predictions introduced by variations in tissue preparation, digital scanners, and the existence of unusual prostate tissue that the model was not exposed to during training.
Why did you choose to study this particular area?
Since this cancer is so common, we need to reduce prostate cancer mortality but also take better care of the men who suffer due to overdiagnosis and overtreatment of low-risk cancer. Aside from the implications for patients, there are significant savings to be made in the healthcare system if we know who to treat and when.
What do you think should be done moving forward in this research area?
Recent studies have shown that evaluation using MRI alone is currently not an option to provide safe monitoring of disease progression in active surveillance. The need for additional biomarkers and clinical variables that can be combined with MRI evaluation, as well as further standardization of reporting of MRI has been identified as key points to address.
There is a lot to be done moving forward to improve the precision and robustness of the performance of the AI model. Our research group is currently aiming to incorporate a couple of key areas into the model architecture: scanner calibration, scaling up the amount of training data, improved algorithms, modeling of morphological heterogeneity, and implementation of automated quality control into the prediction algorithm to make sure that the model only makes predictions on the data it was designed to handle.
Doctoral thesis
Henrik Olsson. Karolinska Institutet (2023), ISBN: 978-91-8016-912-7