Novel deep learning-based models for cancer histopathology image analysis
Histopathological evaluation of tumour specimens has long been essential in diagnosing breast cancer and guiding clinical decision-making. However, one of the key challenges in routine diagnostics include the inter-observer and inter-lab variabilities present in the assessment of prognostic markers that could lead to under- and over-treatment of patients.
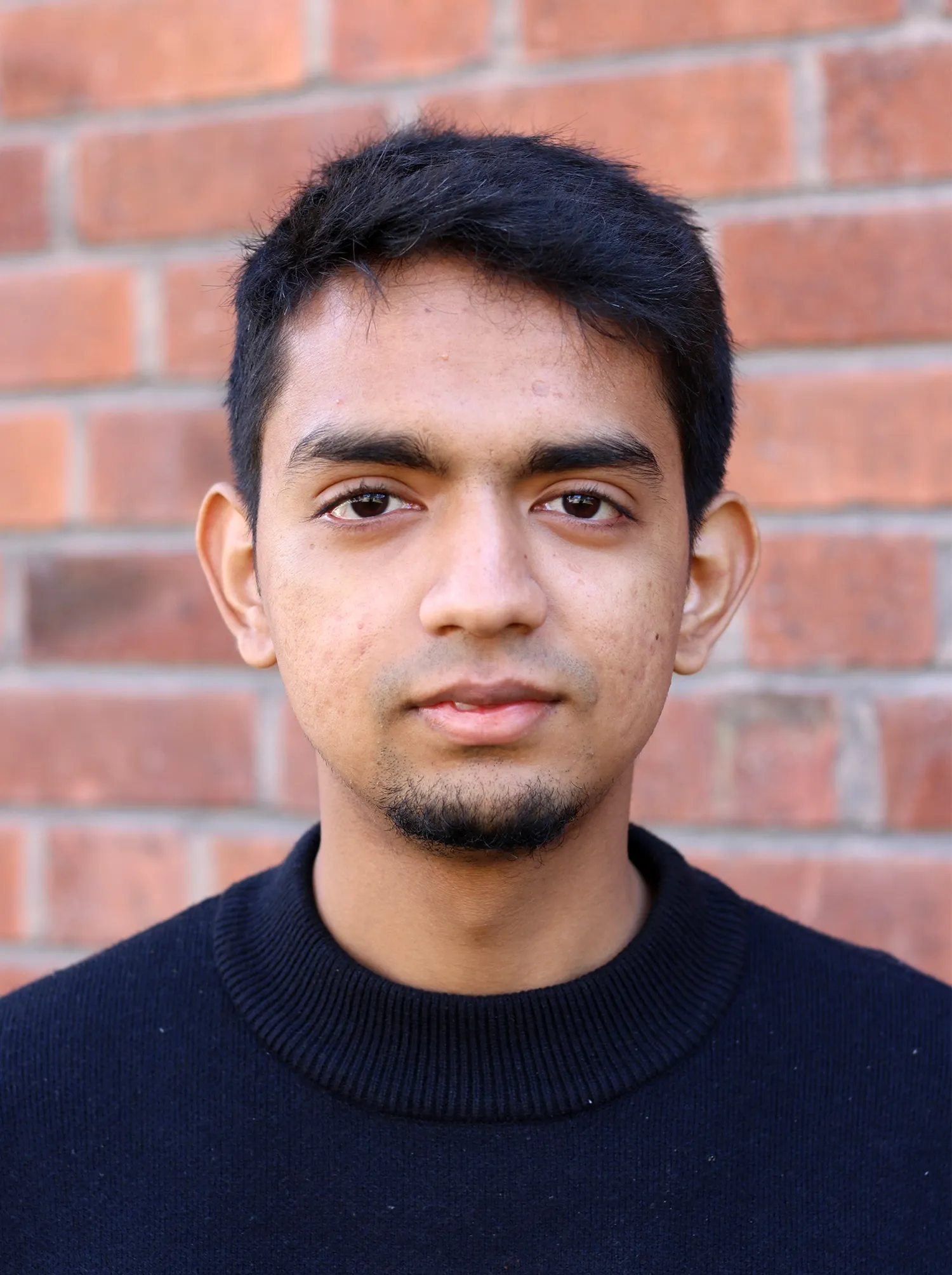
With the current ongoing digitization of pathology labs, it has enabled the advancement of computational pathology which has shown the potential to improve both routine and precision diagnostics and offer decision support to both pathologist and treating physicians to improve breast cancer care.
Deep learning falls under the broader umbrella of Artificial Intelligence (AI) that has shown potential in advancing beyond traditional pathology by improving risk assessment, prognosis prediction, and response-to-treatment predictions. This approach, known as AI-based precision pathology, offers new possibilities for better patient care.
In his thesis, Abhinav Sharma at the Department of Medical Epidemiology and Biostatistics has developed and validated deep learning-based models for AI- based precision pathology tasks to improve breast cancer diagnosis using routinely stained tumour tissue specimens.
What are the most important results in your thesis?
“My thesis includes four different studies and without getting too technical, here are some of my key findings: In my first study, we developed and validated a deep learning-based model (predGrade) that mimics the clinical histogical grade, for classifying invasive breast cancer into three grades based on H&E-stained whole slide images (WSIs). The model showed potential in reducing inter-observer and inter-lab variability, offering a more reproducible and robust clinical decision support tool for breast cancer histological grading. In the second study, we validated an AI-based solution, Stratipath Breast, used for risk stratification in breast cancer, in two independent hospital sites in Sweden. In this retrospective validation study, Stratipath Breast could significantly improve prognostic risk stratification for intermediate-risk breast cancer patients, which can further improve better assignment of adjuvant chemotherapy in such patients and avoid under- and over-treatment of the patients. In study III, we introduced a methodology called the Wsi rEgion sElection approach (WEEP) to spatially interpret the deep learning-based weakly supervised models. This methodology can provide insights into decision making of such AI models that can be useful in both research and diagnostic applications. In study IV, we developed a deep learning-based multi-stain prognostic risk score prediction model using routinely stained WSIs for breast cancer patients. We saw an improvement in prognostic risk score prediction when using the combination of local and spatial alignment of multiple stains in comparison to individual stains that can potentially provide a solution for better risk-stratification of breast cancer patients.”
What do you think should be done in future research?
“I have an interdisciplinary background in bioengineering and have always been passionate about working at the intersection of biology and technology. Recent advancements in applying artificial intelligence to healthcare, particularly in improving diagnostics and providing personalized treatments for cancer patients, have captured my attention.”
Doctoral thesis
“Development and validation of novel deep learning- based models for cancer histopathology image analysis”
Abhinav Sharma. Stockholm: Karolinska Institutet (2025), ISBN: 978-91-8017-843-3