Machine learning methods for precision medicine
In precision medicine, predicting the risk of an event during a specific period may help, for example, to identify patients that need early preventive treatment. Modern machine learning (ML) techniques are therefore ideal for building these predictions.
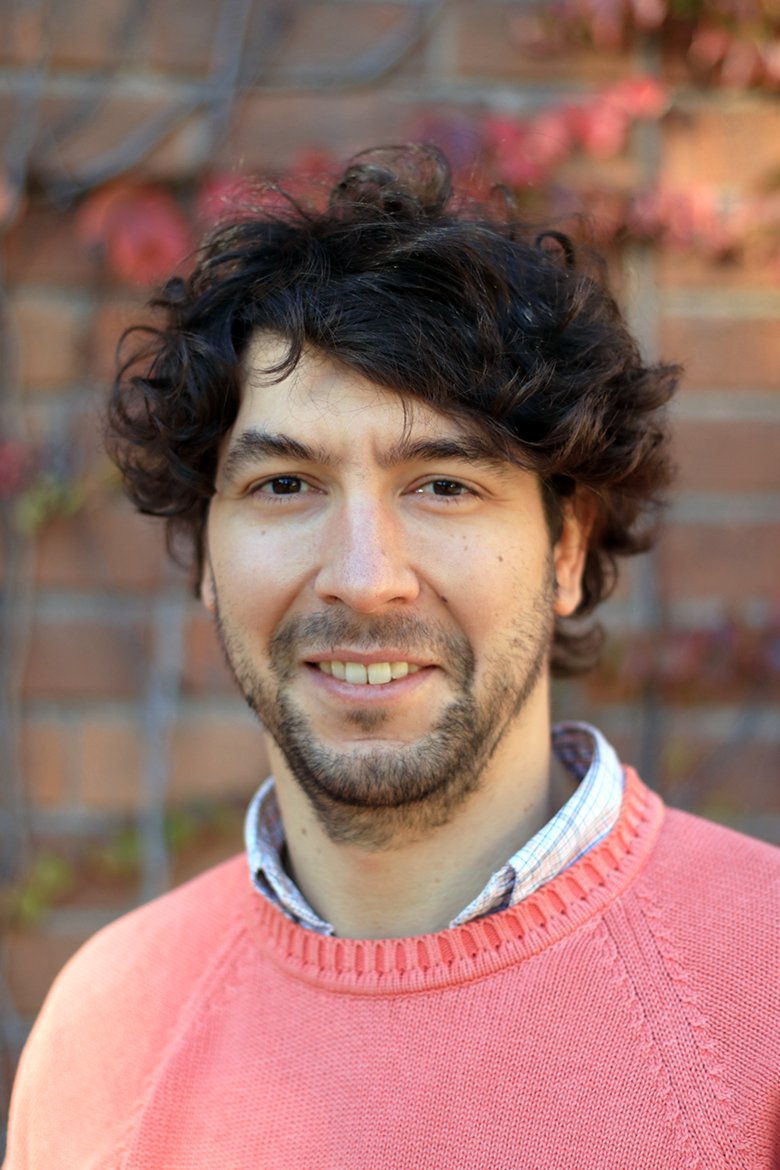
When it comes to medical datasets, they often suffer from right-censoring of the outcome of interest posing an obstacle to the direct applicability of ML algorithms. An observation is said to be right-censored if the patient was alive at study termination or was lost to follow-up at any time during the study.
In his thesis, Pablo Gonzalez Ginestet at the Department of Medical Epidemiology and Biostatistics worked to develop and advance methods for prediction models based on machine learning algorithms in settings of right-censoring, and in some settings also including competing risks.
What are the most important results in your thesis?
–The most important result is that the proposed methods allow building a risk prediction model accounting for censored observations using one or a combination of ML algorithms resulting in a better or similar predictive accuracy to methods that already account for censoring.
Why did you choose to study this particular area?
–I was interested in machine learning methods and my supervisor introduced me to this area of work, which is to find ways to adapt ML methods to settings where the dataset suffer from right-censoring and one does not want to exclude the censored observations from the analysis.
What do you think should be done moving forward in this research area?
–It would be interesting to extend the methodology in settings of interval censoring that occurs in studies that entail periodic follow-up such as in trials of acquired immune deficiency syndrome (AIDS) where the blood testing to determinate AIDS onset is performed periodically.
Doctoral thesis
“Machine learning methods for precision medicine.”
Pablo Gonzalez Ginestet. Karolinska Institutet (2022), ISBN: 978-91-8016-633-1