Statistical tools for valid causal inference with fewer assumptions
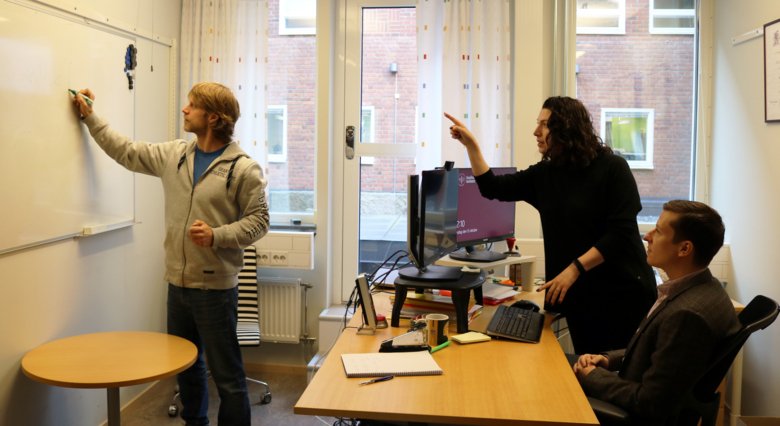
Causal inference is important in medical research to help determine if treatments are beneficial and if natural exposures are harmful. In many settings, data collection makes causal inference difficult without making overly optimistic or idealistic assumptions. In a new article published in the Journal of the American Statistical Association, researchers at Karolinska Institutet develop new statistical methods to make causal inference possible in some settings without making such assumptions.
The authors Erin Gabriel, Michael Sachs and Arvid Sjölander at the Department of Medical Epidemiology and Biostatistics, describe in the new paper how these methods can be used and interpreted.
New tools that can be applied in a variety of different research settings
Randomized trials are a type of experiment where groups of volunteers are randomly assigned to get a new medicine or not, and then a comparison is made between the two randomly assigned groups to assess the effect of the randomized medicine on the survival, infection, or well-being of the patients. Unlike new medicines, there are many things that cannot be assigned randomly to volunteers, such as smoking and asbestos exposure, or that could be randomized, but are most often studied in observational studies, such as red wine and fruit consumption.
In these settings, the effect of an exposure can be difficult to determine because other factors may influence both the exposure of interest and the outcome. For example, living in Sweden is associated with lower mortality and higher consumption of cloudberries than Hungary, so looking for the effect of cloudberries on mortality in a group that included people from both Hungary and Sweden may lead a researcher to believe that cloudberries reduce mortality.
Statistical methods developed using a novel approach
Although there are many tools for dealing with measured factors, such as country of residence, to allow for the testing and estimation of such effects, all of these methods require that a researcher is willing to guess about all other factors they have not measured. The work presented here uses math, logic and statistics to relax the need for this guessing and, rather than giving a single value of the effect, gives a range of possible effect sizes. Although some researchers have developed similar methods, the methods are very few and are specific to the type of data and how the data were collected. Erin Gabriel and her colleagues develop new methods to allow for a much larger number of data collection styles, many of which are very common in Sweden due to the registers.
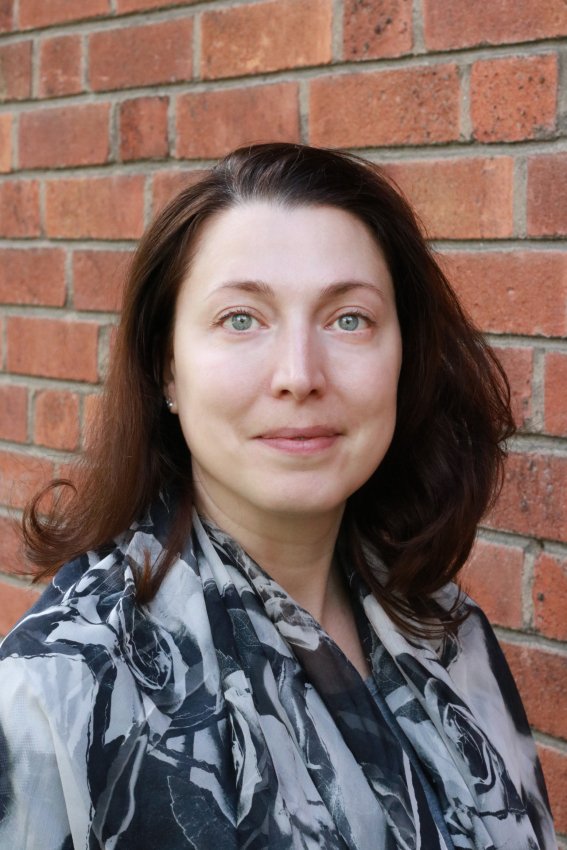
“These statistical methods, which are easy to implement, may help in many settings where causal inference is threatened by unmeasured confounding and/or selection bias,” says first author Erin Gabriel.
The authors hope that their tools will be used by researchers around the world to help them make decisions without having to guess about unmeasured factors in their data. In their ongoing and future work, they aim to build and describe new statistical tools that can be used in imperfect clinical trials.
Publication
Title: Causal bounds for outcome-dependent sampling in observational studies
Authors: Erin E Gabriel, Michael C Sachs, Arvid Sjölander
Journal: Journal of the American Statistical Association
doi: https://doi.org/10.1080/01621459.2020.1832502
arXiv link: https://arxiv.org/abs/2002.10519